Individuals don’t all the time want one other human being to expertise a way of connection. The deep emotional bonds many individuals have with their pets proves this. (So may the recognition of the Pet Rock within the Seventies however that’s simply hypothesis.) Even Hyperlink in The Legend of Zelda had an inanimate companion: his trusty sword (see Determine 9.1).
Article Continues Beneath
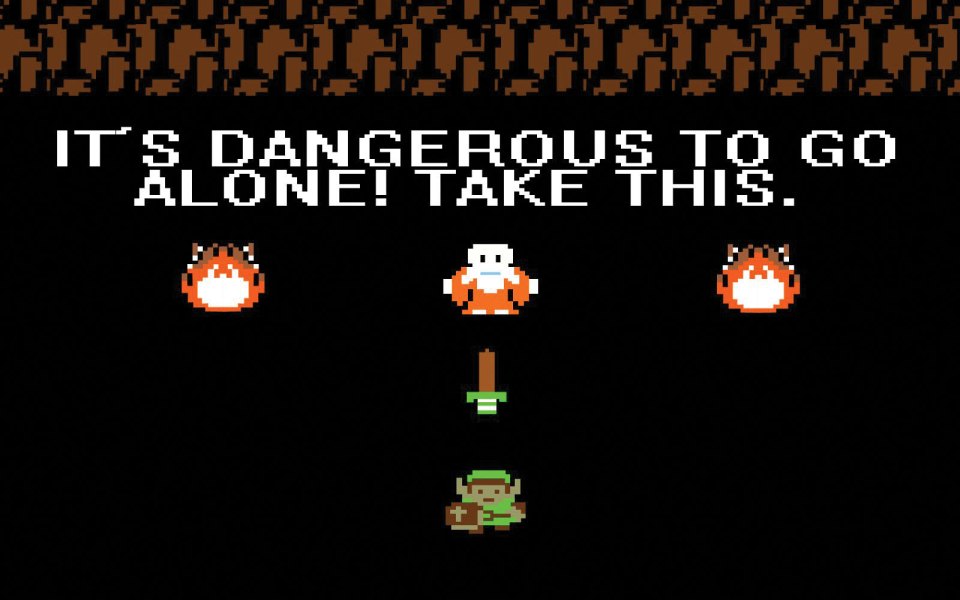
It’s additionally potential for individuals to really feel that sense of connection within the context of habits change with out having direct relationships with others. By constructing your product in a manner that mimics a few of the traits of a person-to-person relationship, you may make it potential to your customers to really feel linked to it. It’s potential to coax your customers to fall not less than a little bit bit in love together with your merchandise; for those who don’t imagine me, attempt to get an iPhone person to modify working programs.
It’s not nearly actually liking a product (though you undoubtedly need customers to actually like your product). With the appropriate design components, your customers may embark on a significant bond together with your know-how, the place they really feel engaged in an ongoing, two-way relationship with an entity that understands one thing essential about them, but is recognizably non–human. It is a true emotional attachment that provides not less than a few of the advantages of a human-to-human relationship. This sort of connection can assist your customers have interaction extra deeply and for an extended time period together with your product. And that ought to in the end assist them get nearer to their habits change objectives.
Amp Up the Anthropomorphization#section2
Individuals can forge relationships with non–people simply due to a course of known as anthropomorphization. To anthropomorphize one thing means to impose human traits on it. It’s what occurs if you see a face within the array of shapes on the appropriate aspect in Determine 9.2, or if you keep it up an prolonged dialog together with your cat.[1]
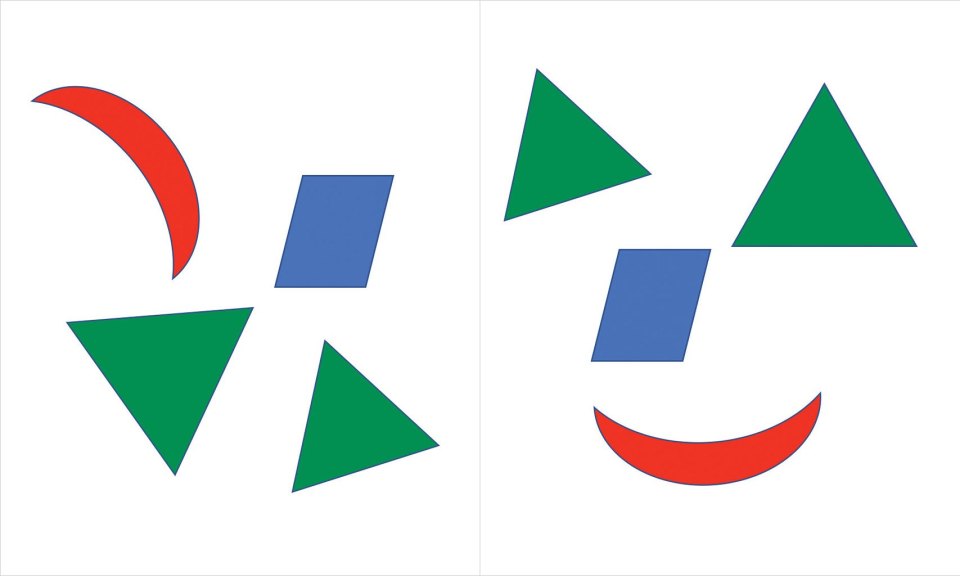
Individuals will discover the human qualities in shapes that barely resemble a face, however you possibly can assist velocity that course of alongside by intentionally imbuing your product with bodily or character options that resemble individuals. Voice assistants like Siri, Cortana, and Alexa, for instance, are simply perceived as human-like by customers because of their capacity to hold on a dialog very similar to a (considerably single-minded) particular person.
Granted, virtually no one would mistake Alexa for an actual particular person, however her human traits are fairly convincing. Some analysis means that kids who develop up round these voice assistants could also be much less well mannered when asking for assist, as a result of they hear adults make calls for of their gadgets with out saying please or thanks. When you’re asking Siri for the climate report and there are little ones in earshot, think about including the different magic phrases to your request.
So, if you would like individuals to anthropomorphize your product, give it some human traits. Assume names, avatars, a voice, and even one thing like a catchphrase. These particulars will put your customers’ pure anthropomorphization tendencies into hyperdrive.
Every thing Is Private#section3
One factor people do nicely is personalization. You don’t deal with your father or mother the identical manner you deal with your partner the identical manner you deal with your boss. Every interplay is totally different based mostly on the id of the particular person you’re interacting with and the historical past you may have with them. Expertise can supply that very same sort of individualized expertise as one other approach to mimic individuals, with numerous different advantages.
Personalization is the Swiss Military Knife of the habits change design toolkit. It may possibly assist you to craft applicable objectives and milestones, ship the appropriate suggestions on the proper time, and supply customers significant decisions in context. It may possibly additionally assist forge an emotional connection between customers and know-how when it’s utilized in a manner that helps customers really feel seen and understood.
Some apps have beautiful interfaces that allow customers choose colours or background photos or button placements for a “customized” expertise. Whereas a lot of these options are good, they don’t scratch the itch of belonging that true personalization does. When personalization works, it’s as a result of it displays one thing important in regards to the person again to them. That doesn’t imply it needs to be extremely deep, but it surely does have to be considerably extra significant than whether or not the person has a pink or inexperienced background on their residence display screen.
Personalised Preferences#section4
Throughout onboarding or early in your customers’ product expertise, enable them to personalize preferences that may form their experiences in significant methods (not simply colour schemes and dashboard configurations). For instance, Fitbit asks individuals their most well-liked names, after which greets them periodically utilizing their choice. Equally, LoseIt asks customers throughout setup in the event that they take pleasure in utilizing information and know-how as a part of their weight reduction course of (Determine 9.3). Customers who say sure are given a possibility to combine trackers and different gadgets with the app; customers who say no are funneled to a handbook entry expertise. The person expertise modifications to honor one thing particular person in regards to the person.
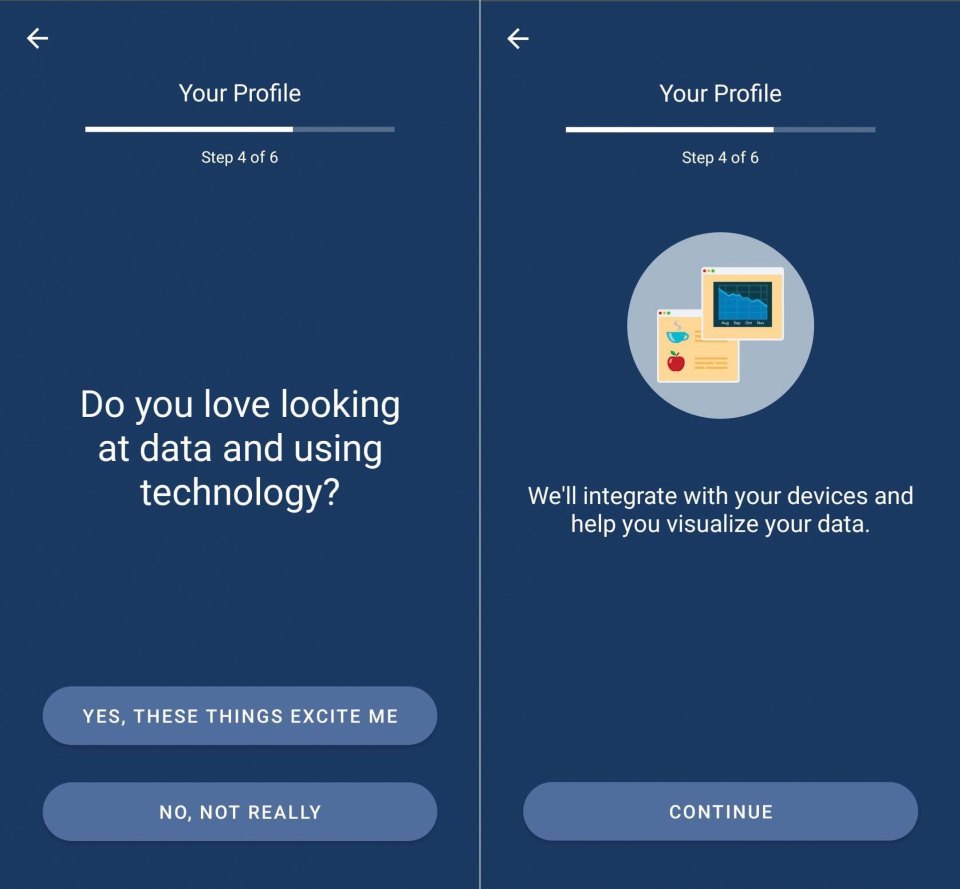
When you can, recall again to historical occasions when Fb launched an algorithmic type of posts within the newsfeed. Fb customers are typically upset anytime there’s a dramatic change to the interface, however their frustration with this one has endured, for one core motive: Fb to today reverts to its personal sorting algorithm as a default, even when a person has chosen to prepare content material by date as a substitute. This repeated insistence on their choice over customers’ makes it much less seemingly that customers will really feel “seen” by Fb.[2]
Personalised Suggestions#section5
When you’ve ever shopped on-line, you’ve most likely obtained customized suggestions. Amazon is the quintessential instance of a advice engine. Different generally encountered customized suggestions embody Fb’s “Individuals You Might Know” and Netflix’s “Prime Picks for [Your Name Here].” These instruments use algorithms that recommend new objects based mostly on information about what individuals have completed up to now.
Suggestion engines can comply with two primary fashions of personalization. The primary one relies on merchandise or objects. Every merchandise is tagged with sure attributes. For instance, for those who have been constructing a exercise advice engine, you may tag the merchandise of “bicep curls” with “arm train,” “higher arm,” and “makes use of weights.” An algorithm may then choose “triceps pulldowns” as an identical merchandise to advocate, because it matches on these attributes. This sort of advice algorithm says, “When you appreciated this merchandise, you’ll like this comparable merchandise.”
The second personalization mannequin relies on individuals. Individuals who have attributes in widespread are recognized by a similarity index. These similarity indices can embody tens or tons of of variables to exactly match individuals to others who’re like them in key methods. Then the algorithm makes suggestions based mostly on objects that lookalike customers have chosen. This advice algorithm says, “Individuals such as you appreciated these things.”
In actuality, most of the extra subtle advice engines (like Amazon’s) mix the 2 varieties of algorithms in a hybrid method. They usually’re efficient. McKinsey estimates that 35% of what Amazon sells and 75% of what Netflix customers watch are advisable by these engines.
Generally what seem like customized suggestions can come from a a lot easier type of algorithm that doesn’t take a person person’s preferences into trương mục in any respect. These algorithms may simply floor the recommendations which can be hottest amongst all customers, which isn’t all the time a horrible technique. Some issues are in style for a motive. Or suggestions may very well be made in a set order that doesn’t rely upon person traits in any respect. This seems to be the case with the Fabulous habits change app that gives customers a sequence of challenges like “drink water,” “eat a wholesome breakfast,” and “get morning train,” no matter whether or not these behaviors are already a part of their routine or not.
When advice algorithms work nicely, they can assist individuals on the receiving finish really feel like their preferences and wishes are understood. Once I browse the playlists Spotify creates for me, I see a number of facets of myself mirrored. There’s a playlist with my favourite 90s alt-rock, one with present artists I like, and a 3rd with a few of my favourite 80s music (Determine 9.4). Amazon has an identical capacity to efficiently extrapolate what an individual may like from their searching and buying historical past. I used to be all the time amazed that despite the fact that I didn’t purchase any of my kitchen utensils from Amazon, they by some means discovered that I’ve the pink KitchenAid line.
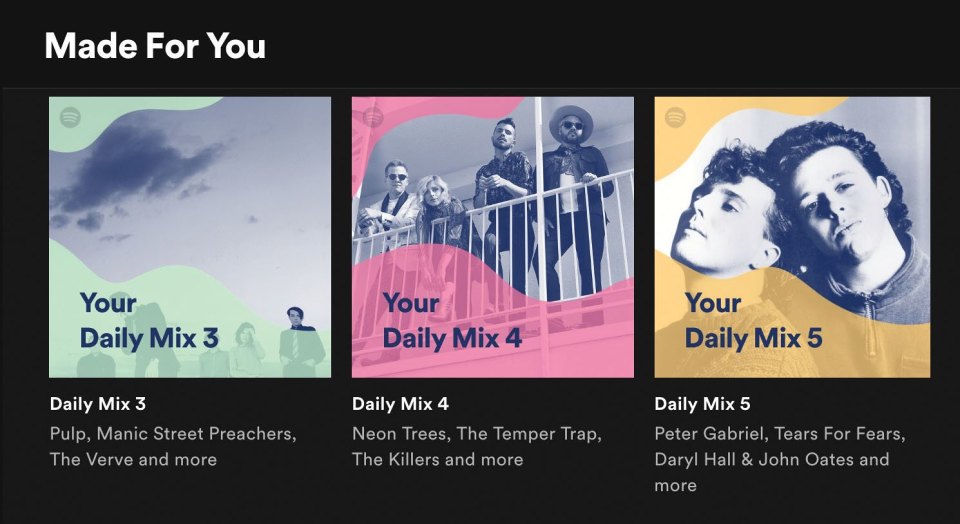
A threat to this method is that suggestions may develop into redundant because the database of things grows. Retail merchandise are a simple instance; for a lot of objects, as soon as individuals have purchased one, they seemingly don’t want one other, however algorithms aren’t all the time sensible sufficient to cease recommending comparable purchases (see Determine 9.5). The identical type of repetition can occur with habits change applications. There are solely so many alternative methods to set reminders, for instance, so sooner or later it’s a good suggestion to cease bombarding a person with recommendations on the subject.
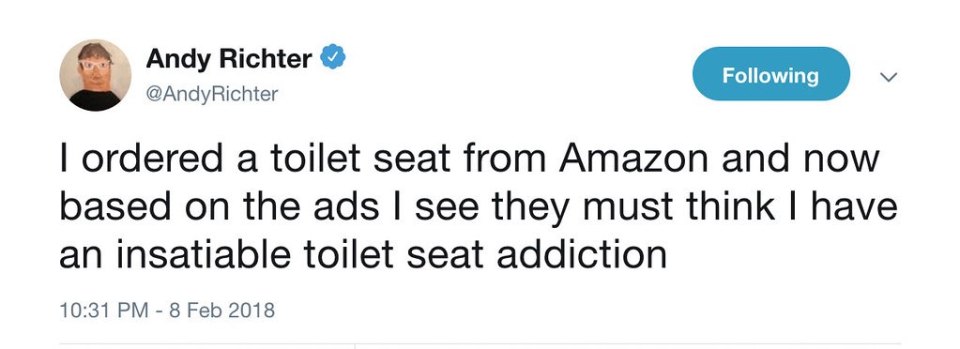
Don’t Be Afraid to Study#section7
Information-driven personalization comes with one other set of dangers. The extra you realize about customers, the extra they count on you to offer related and correct recommendations. Even the neatest know-how will get issues improper typically. Give your customers alternatives to level out in case your product is off-base, and regulate accordingly. Not solely will this enhance your accuracy over time, however it would additionally reinforce your customers’ emotions of being cared for.
Alfred was a advice app developed by Intelligent Sense to assist individuals discover new eating places based mostly on their very own preferences, in addition to enter from their social networks. Considered one of Alfred’s mechanisms for gathering information was to ask customers to verify which eating places they appreciated from an inventory of prospects (see Determine 9.6). Explicitly together with coaching within the expertise helped Alfred make higher and higher suggestions whereas additionally giving customers the chance to chalk errors as much as a necessity for extra coaching.[3]

Having a mechanism for customers to exclude a few of their information from an algorithm will also be useful. Amazon permits customers to point which objects of their buy historical past ought to be ignored when making suggestions—a function that is useful for those who purchase items for family members whose tastes are very totally different from yours.
On the flip aspect, intentionally throwing customers a curve ball is an effective way to be taught extra about their tastes and preferences. Over time, algorithms are more likely to develop into extra constant as they get higher at sample matching. Including the occasional mold-breaking suggestion can forestall boredom and higher trương mục for customers’ quirks. Simply because somebody loves meditative yoga doesn’t imply they don’t additionally like going mountain biking now and again, however most advice engines received’t be taught that as a result of they’ll be too busy recommending yoga movies and mindfulness workouts. Once in a while add one thing into the combination that customers received’t count on. They’ll both reject it or give it a whirl; both manner, your advice engine will get smarter.
Personalised Teaching#section8
In some unspecified time in the future, suggestions within the context of habits change might develop into one thing extra sturdy: an precise customized plan of motion. When suggestions develop out of the “you may additionally like” section into “right here’s a sequence of steps that ought to give you the results you want,” they develop into a little bit extra sophisticated. As soon as a bunch of customized suggestions have some type of cohesiveness to systematically information an individual towards a purpose, it turns into teaching.
Extra deeply customized teaching results in more practical habits change. One research by Dr. Vic Strecher, whom you met in Chapter 3, confirmed that the extra a smoking cessation teaching plan was customized, the extra seemingly individuals have been to efficiently give up smoking. A follow-up research by Dr. Strecher’s crew used fMRI know-how to find that when individuals learn customized info, it prompts areas of their mind related to the self (see Determine 9.7). That’s, individuals understand customized info as self-relevant on a neurological degree.

That is essential as a result of persons are extra more likely to bear in mind and act on related info. In order for you individuals to do one thing, personalize the expertise that exhibits them how.
From a sensible perspective, customized teaching additionally helps overcome a typical barrier: Individuals don’t need to spend so much of time studying content material. In case your program can present solely probably the most related objects whereas leaving the generic stuff on the reducing room flooring, you’ll supply extra concise content material that individuals may very well learn.